ABC Easy as 123: A Blind Counter for Exemplar-Free
Multi-Class Class-agnostic Counting
ABC123
Class-agnostic counting methods enumerate objects of an arbitrary class, providing tremendous utility in many
fields.
Prior works have limited usefulness as they require either a set of examples of the type to be counted or that the
query image contains only a single type of object. A significant factor in these shortcomings is the lack of a
dataset to properly address counting in settings with more than one kind of object present.
To address these issues, we propose the first Multi-class, Class-Agnostic Counting dataset (MCAC) and A Blind
Counter (ABC123), a method that can count multiple types of objects simultaneously without using examples of type
during training or inference.
ABC123 introduces a new paradigm where instead of requiring exemplars to guide the enumeration, examples are found
after the counting stage to help a user understand the generated outputs.
We show that ABC123 outperforms contemporary methods on MCAC without needing human in-the-loop annotations. We
also show that this performance transfers to FSC-147, the standard class-agnostic counting dataset.
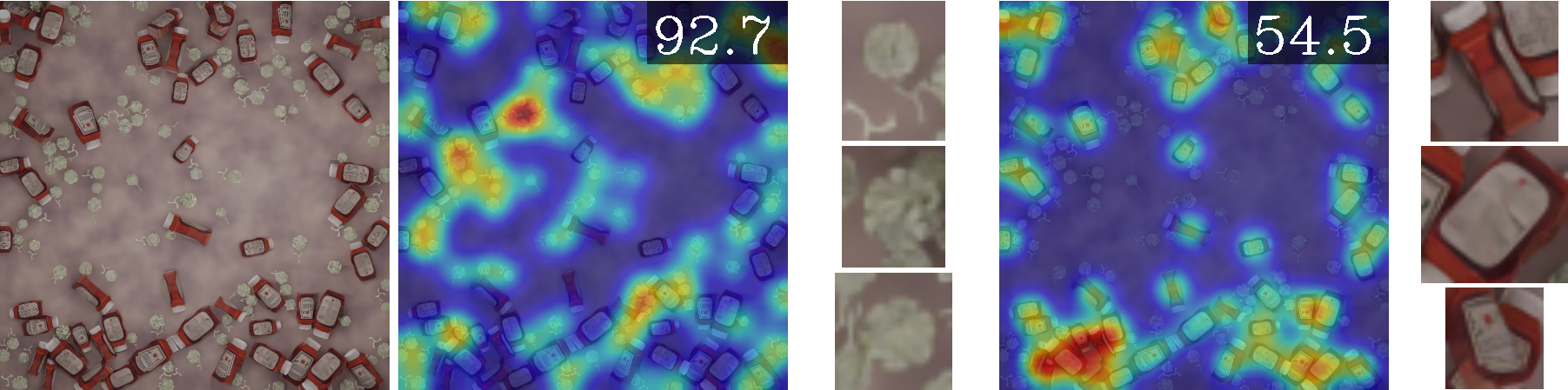
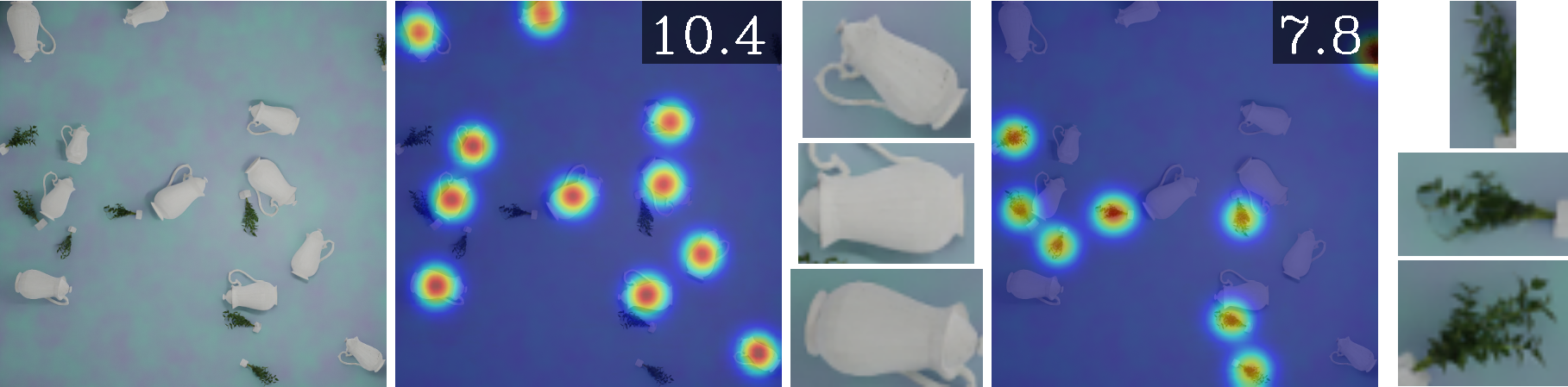
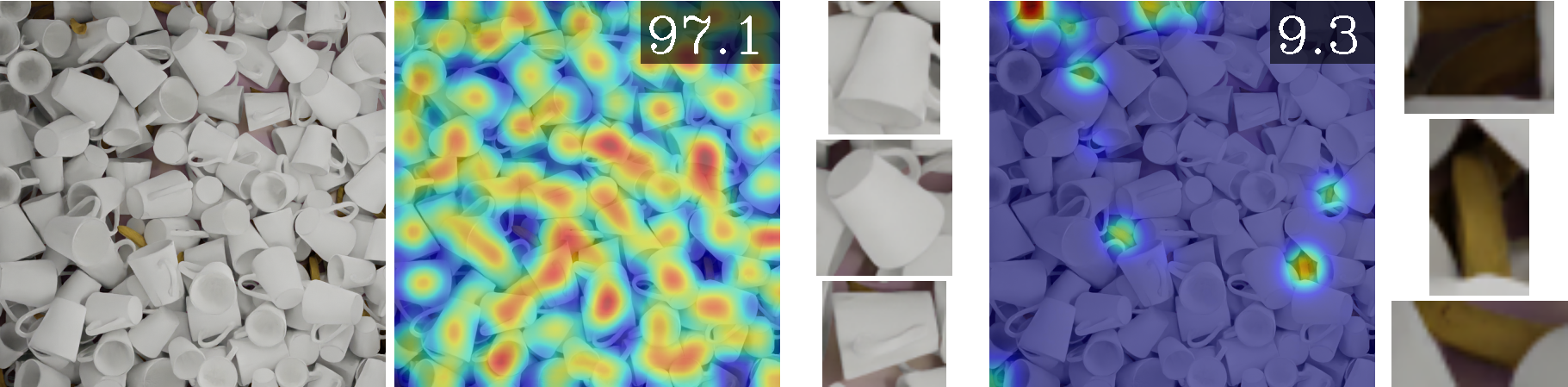


MCAC
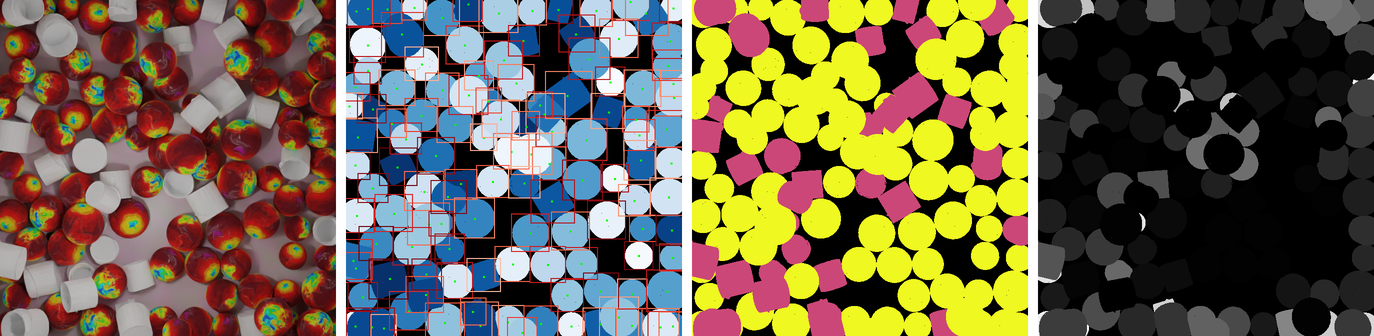
MCAC is the first multi-class class-agnostic counting dataset. For details on MCAC see its project page.
Benchmark Results
Val | Test | ||||||||
Method | Shots | MAE | RMSE | NAE | SRE | MAE | RMSE | NAE | SRE |
Mean | N/A | 39.87 | 53.56 | 3.07 | 11.40 | 42.67 | 59.68 | 2.79 | 10.93 |
Median | N/A | 36.25 | 58.15 | 1.51 | 6.70 | 39.81 | 65.36 | 1.38 | 6.73 |
FamNet | 3 | 24.76 | 41.12 | 1.12 | 6.86 | 26.40 | 45.52 | 1.04 | 6.87 |
BMNet | 3 | 15.83 | 27.07 | 0.71 | 4.97 | 17.29 | 29.83 | 0.75 | 6.08 |
CounTR | 3 | 15.07 | 26.26 | 0.63 | 4.79 | 16.12 | 29.28 | 0.67 | 5.71 |
ABC123 | 0 | 8.96 | 15.93 | 0.29 | 2.02 | 9.52 | 17.64 | 0.28 | 2.23 |
Citation
@article{hobley2023abc, title={ABC Easy as 123: A Blind Counter for Exemplar-Free Multi-Class Class-agnostic Counting}, author={Hobley, Michael and Prisacariu, Victor}, journal={Proceedings of the European Conference on Computer Vision}, year={2024}}